Stats miscellany (3)
Here are some lukewarm takes on recent events in statistics and associated domains. At risk of jinxing the endeavor, I’ll continue trying to make this a semi-regular feature.
Drug ratings patterns
In a characteristically insightful and terrifying post on Slate Star Codex, Scott Alexander notices some disturbing patterns in patient reviews of prescription drugs.
Across the class of antidepressants, doctor ratings and patient ratings of these drugs are anticorrelated, with the relationship being nonsignificant. Although my inclination would be to hedge a bit more, I tend toward Alexander’s interpretation that “at best, doctor reviews are totally uncorrelated with patient consensus”. Alexander also does some more qualitative digging to suggest that “the more patients like a drug, the less likely it is to be prescribed”.
Further, patient ratings of antidepressants are negatively correlated with drug release data, with this relationship being significant. (Alexander should probably flip the axes on that graph.)
There’s a grand narrative that explains some of these patterns, involving the drug class of MAOIs being supplanted by the generally better tolerated but less effective SSRIs and their brethren. Additionally there are lots of statistical confounders and complications to be checked and accounted for that might explain away the observed patterns. But if they hold up, they point to huge mechanism design problem to be solved in nudging the pharmaceutical-medical system to developing and promoting effective medications.
Measurement
Andrew Gelman claims that “the #1 neglected topic in statistics is measurement”. Alexander’s post would be a great case study in this, with its (perhaps unavoidable) reliance on subjective (self-)reports from populations of patients with conditions that might affect this reporting and from populations of doctors under an intense marketing onslaught designed to shape their opinions.
The paper “Race and Gender Discrimination in Bargaining for a New Car” by Ian Ayres and Peter Siegelman gives a vibrant example of how much care and effort might go into measurement:
The paired audit technique is designed to eliminate as much intertester variation as possible, and thus to insure that differences in outcomes (such as prices quoted) reflect differences in dealer rather than tester behavior. We began by choosing testers according to the following criteria:
- Age: All testers were between 28 and 32 years old.
- Education: All testers had 3–4 years of postsecondary education.
- Attractiveness: All testers were subjectively chosen to have average attractiveness.
The testers also displayed similar indicia of economic class. Besides volunteering that they did not need financing, all testers wore similar “yuppie” sportswear and drove to the dealership in similar rented cars.
The script governed both the verbal and nonverbal behavior of the testers, who volunteered very little information and were trained to feel comfortable with extended periods of silence.
Insufficiency of sufficient statistics
A statistician’s first instinct in being exposed to a new experimental situation or dataset is to find a model that captures the system’s complexity while allowing for data reduction down to a manageable collection of sufficient statistics. This allows for less cognitive and record-keeping effort without any loss of statistical capability (with respect to the chosen model).
However, Andrea Montari describes, in “Computational Implications of Reducing Data to Sufficient Statistics”, some situations where this reduction can have grievous computational consequences. The argument that raises the possibility of the problem is straightforward and almost obvious in hindsight:
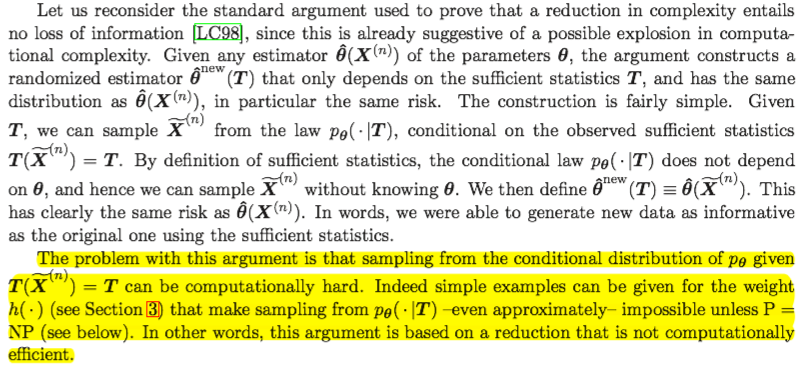
The demonstration that this is a practical problem is the rest of the paper, and I’m still in the middle of reading it.
Data Science from Scratch
Joel Grus announces the release of his book Data Science from Scratch: First Principles with Python and I’m taking a look through it. A full review might follow soon.
I don’t think I’ll necessarily learn very much at the object-level from it, but I’m interested in the learning about the meta-level perspective that Grus describes here:
Over the years, I’ve trained a number of data scientists. While not all of them have gone on to become world-changing data ninja rockstars, I’ve left them all better data scientists than I found them. And I’ve grown to believe that anyone who has some amount of mathematical aptitude and some amount of programming skill has the necessary raw materials to do data science. All she needs is an inquisitive mind, a willingness to work hard, and this book. Hence this book. (p. xiii)
This persepctive seems important not just pedagogically, but in identifying and creating tools and methods that data scientists will find useful and usable in their work.
Alien brains
Peter Watts drops a fiblet titled “Colony Creature”. Through a fictional brain-brain interface (which is sketched as the intercommunication of two brain-computer interfaces that don’t quite speak the same language), Watts tries to answer the question “what’s it like to be an octopus?”:
“Those arms.” His Adam’s apple bobbed in his throat. “Those fucking crawly arms. You know, that thing they call the brain— it’s nothing, really. Ring of neurons around the esophagus, basically just a router. Most of the nervous system’s in the arms, and those arms… every one of them is awake…”
Like so much of Watts’ work, it’s a simultaneously academic and chilling exploration of how tightly cognitive function is bound to cognitive form.